Object-based image analysis software can help to identify and map marine debris on remote beaches. By Mary Jo Wagner
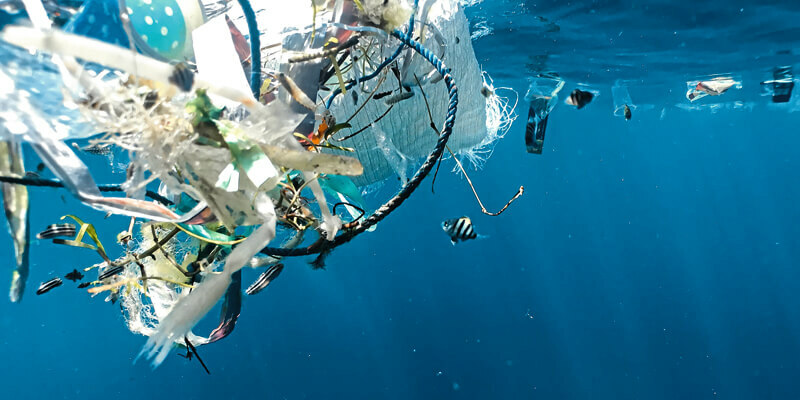
“Seeing is believing” can be a compelling and persuasive tool to catalyse change. In the case of the world’s oceans and seas, the layers of garbage bobbing on their surfaces and washing up on shores cannot be unseen – sometimes there is so much of it, seeing it defies belief. But that visibility has made it easier to unify public and private interest in studying, innovating and implementing strategies to resolve the issue of marine litter.
One key step to addressing our “plastics problem” is to understand the characteristics of the debris itself: where it is, what type it is and how much there is. The sheer vastness of the target area makes it nearly impossible to accurately monitor, log and model the presence of plastics, metals and fishing gear floating in the water, so efforts have often turned inland to beaches and coastlines. Typically, that has required organised beach surveys with teams of volunteers manually recording found debris and cleaning it up. Although beach clean-ups help, they are costly and are mostly concentrated near population centres, leaving scattered garbage on more remote beaches untouched.
However, advancements in drone technology, robotics, machine learning and object-based image analysis (OBIA) software are helping plot a path to a more efficient and effective approach to minimising sea litter. OBIA technology, in particular, is showing great promise for automating the detection and identification of plastics and fish nets floating on the surface and cluttering beaches.
“OBIA, with remote sensing data such as drone imagery, is a powerful tool for detecting and classifying unique types of debris,” says Frank Beuchel, a senior scientist with Akvaplan-niva (APN), a research subsidiary of the Norwegian Institute of Water Research based in Tromsø, Norway. “You can integrate spectral, spatial, textual, and contextual properties in one interlinked framework and the software will analyse a large area and automatically identify and classify litter with high precision in a few minutes. Combining this process with high-resolution drone or satellite imagery would offer a much faster, precise and repeatable method for monitoring and managing beach garbage.”
The prevalence of plastic
As a research and development institute focusing on aquaculture and coastal zone management, APN has led and participated in several projects that investigate the distribution of plastics and their effects. In 2019, they responded to a call from the Research Council of Norway (RCN) for innovations that targeted terrestrial pollution – specifically, methodologies that would indicate where marine debris can be found, how much there is and which type.
“There has been growing concern about the prevalence of marine litter in northern Norway for many years, since studies began showing a dominance of plastics and a high proportion of fishing-associated debris in Arctic waters,” says Beuchel. “There are still major knowledge gaps about where litter is distributed throughout the Norwegian, Barents, and Kara seas, and in the High Arctic in general. An automated debris-mapping approach would be an effective solution for monitoring beaches and targeting clean-ups. We saw the RCN’s interest as the perfect opportunity to develop a methodology with OBIA.”
That same year, the Mapping Marine Litter in the Norwegian and Russian Arctic Seas (MALINOR) project was launched.
MALINOR
A multinational Norway-Russia project with 13 partners, MALINOR’s objective is to map and describe the types of marine litter found along shorelines of the Norwegian Sea, the Barents Sea, the Arctic Ocean and the Kara Sea. Using multicopter drones, optical satellite imagery, a wave glider (ocean drone) and Trimble’s eCognition OBIA software, the project team aims to validate novel technologies for automatically identifying marine debris in both drone and satellite images and mapping their distribution on beaches. Of particular importance is how well the new approach can detect fish nets.
According to a 2019 report by Greenpeace, more than 640,000 tonnes of nets, lines, pots and traps used in commercial fishing are dumped and discarded in the sea every year, the same weight as 55,000 double-decker buses. And once a net is left in the water, it continues to trap fish for as long as three years.
“Lines, nets, buoys and other fishing gear account for up to 50% of all recorded beach litter in Norway,” says Beuchel. “So we were especially interested in creating and assessing an automated solution that could accurately identify and map fish nets and fishing- related gear at different spatial resolutions.”
Sourcing the data
The project team first sampled several remote beaches – each 100m wide – in northern Norway and Russia to quantify coastal macro litter and identify hotspots along the coasts of the Norwegian, Barents and Kara Seas. Dividing each beach into three, 10m-wide transects, each 30 m apart, survey teams from SALT, one of the leading beach-cleaning companies in Norway, photographed, measured and weighed found litter and organised them into eight categories based on size and source/release points.
Sea litter data was also collected from the water using a novel wave glider, an autonomous, unmanned surface vehicle with modular sensors and payloads that can capture data in real time. For MALINOR, a Go-Pro camera was attached a few metres below the glider and captured pictures of the water surface every seven seconds.
In parallel with the ground survey efforts, several drone flights over each area of interest (AOI) collected images with 1cm resolution from an altitude of 50 m. All the photos were then orthorectified and stitched together into seamless mosaics. Four WorldView-3 scenes with 30cm resolution were also acquired.
With source data prepared, the next step was developing the eCognition analysis and classification workflow – the key piece of their mapping solution that could bring better precision and efficiency to the traditionally manual mapping and laborious beach clean-ups.
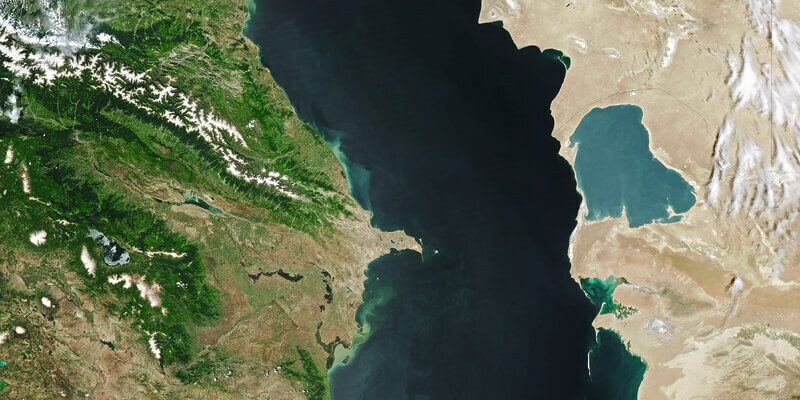
Netting the litter
For this particular work, Jørgensen created three main rule sets – one for the glider, one for aerial drones, and one for satellite data – along with several smaller, customised workflows for each type of litter targeted. Although the glider rule set focused on a simpler task of separating plastics from non-plastics, the drone and satellite rule sets were much more involved.
Predominantly using RGB values, brightness and specific indices such as a Green Vegetation Index and Normalized Soil Index, eCognition moved from one orthomosaic tile to the next and used tiered workflows and a series of segmentation and classification algorithms to distinguish debris from other objects like vegetation and rocks. After sorting these, the software began the process of identifying specific debris types – a hierarchical classification approach that optimises processing efficiency and rule-set transferability.
Using a multi-resolution segmentation algorithm defined by RGB values, the rule set first segmented the data stack into meaningful objects, separating out plastics. User-defined thresholds such as texture, saturation values, and geometry, enabled the software to identify specific debris classes, distinguishing each colour of plastic as well as fish nets. eCognition followed this workflow for each plastic colour — red, blue, white, pink, etc – and for each fish net.
In total, the software delineated nine classes: eight distinct plastics and fish nets for each AOI – classification results that took less than 10 minutes and were 90% accurate. The results were then exported to Esri ArcGIS and QGis for final analysis and mapping.
“The resolution of the aerial drone imagery is high enough that we can initially verify eCognition results ourselves,” says Nils Erik Jørgensen, CTO and owner of TerraNor, a project partner and remote sensing and GIS company based in Roa, Norway. “We found all the debris in the images, from plastics and fish net pieces only a few centimetres in size to larger pieces over 100cm. And it even picked up material that we didn’t readily see ourselves. Some beaches were clean; others had up to 100 pieces more than 1cm large. Most importantly, the software found all the fish nets, a main focus for the project.”
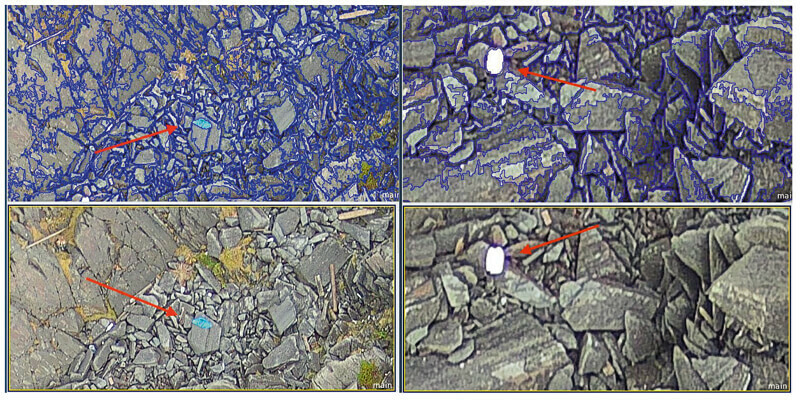
For WorldView imagery, eCognition is delineating objects into the same nine classes using the satellite sensor’s eight channels – panchromatic, multispectral, short-wave infrared. The software first distinguishes water and algae, and then separates out vegetation. Segmenting the images into single, 30cm pixels, it then uses the predefined spectral, textural and geometric thresholds to categorise individual plastics and fish nets.
Although the satellite-based analysis is still ongoing, preliminary outcomes show that eCognition is identifying green and red fish nets with ease. Further validation of both the drone and satellite-based results are being performed by SALT teams who are comparing the eCognition classifications with the ground truth data they collected.
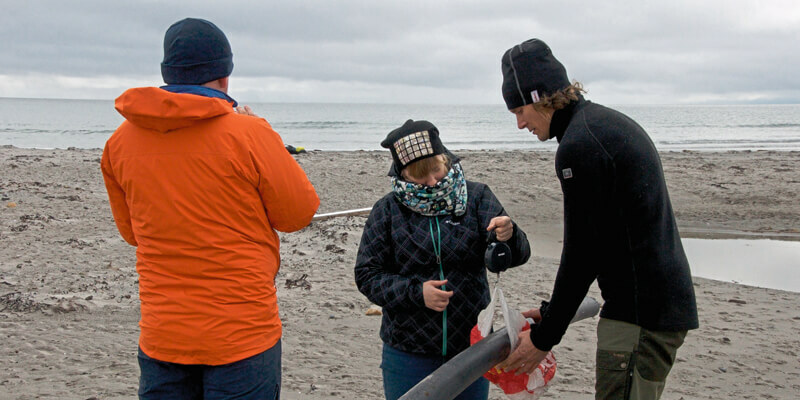
Casting the net further
Since proving the OBIA approach with MALINOR, APN and TerraNor have teamed up again on two other projects designed to automatically identify and map marine debris. Both funded by the Norwegian Retailers’ Environment Fund, the largest private environmental fund in Norway, DIMARC (Detecting, Identifying, and Mapping Plastic in the Arctic) is focused on detecting “ghost gear” – lost or abandoned fishing gear on shorelines – and CRIPTIC (Cleaning Riverine Plastic from a Transboundary Inflow to the Caspian) aims to apply digital methods and community engagement to reduce plastic waste in the Caspian Sea.
Similar to MALINOR, DIMARC project partners are using a wave glider, aerial drones, satellite imagery and eCognition to identify and map plastic litter offshore and onshore in the Norwegian Arctic. For ground truthing, teams from SALT are performing the field work, recording debris found along the beaches in Troms and Finnmark county. Additionally, the team is studying the drift of plastic in the Arctic using a high-resolution ocean model called FVCOM to determine trajectories and potential sources of sea litter as well as where it could wash ashore.
The project results will be shared with local authorities, NGOs, scientists and students to organise onsite training for field survey and clean-up activities, and ideally, raise public awareness and community engagement in combating plastic waste.
Although a multitude of organisations are addressing this pressing marine debris problem, and making significant progress, still more “seeing is believing” may be needed to further catalyse positive change in the treatment of our waters. Innovations such as these can be a powerful tool to help move the needle towards healthier seas.
Mary Jo Wagner is a freelance journalist who's been writing about geospatial technology for more than 25 years ([email protected])