It can BE hard to justify the cost of maintaining 3D data to the same standard as 2D data. Seb Lessware asks if computerised synchronisation might be the way forward
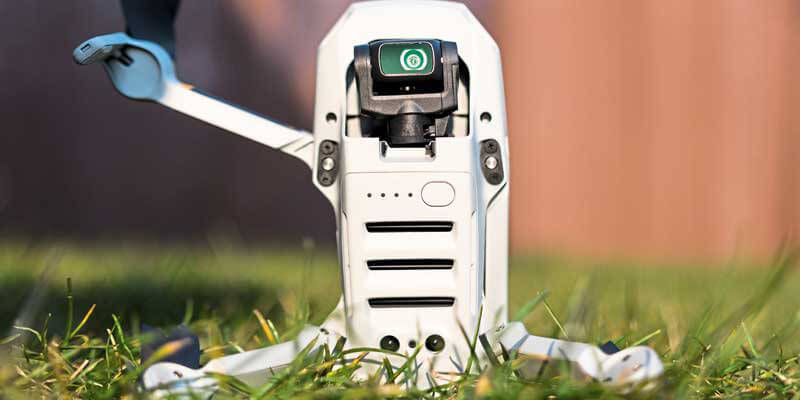
For decades, we have relied on spatial data agencies to provide us with an accurate representation of the natural and man-made landscape. As tools, technologies and standards have evolved, so too has the quality and richness of the spatial data maintained by these organisations.
Whilst the detailed 2D footprint delivered by traditional surveying and production methods has served us all well, users are increasingly looking to 3D data to provide them with in-depth spatial solutions – from urban noise simulations to calculating solar energy capacity and visualising the view, aspect and position of properties for taxation purposes. Indeed, it is already good practice for construction companies to hand over 3D data as part of the ‘as built’ record at the end of a project as part of the increasing adoption of Building Information Modelling (BIM) techniques.
The use cases for Digital Twins and Smart Cities are driving the business case for
capturing national authoritative datasets of 3D data and it is become much more cost-effective to do so with the use of UAVs, airborne LiDAR and other sensors, as well as more traditional photogrammetry or site surveys.
For national authoritative spatial data producers, however, it can be hard to justify the cost and effort of maintaining 3D data to the same rigorous standard as the 2D information for which they are so well-known.
Approaches to managing and maintaining 3D data
Having 3D data that is not synchronised with 2D data – both geometrically and temporally – is bad for users and damaging to the reputation of the data provider, so how to ensure that the two types of data are synchronised and managed cost-effectively?
One thought might be to create 3D data by extruding the 2D data or applying ‘representative’ template 3D shapes but this does not give sufficient detail and accuracy. At best, it provides a quick and crude solution, showing for example, the height of a building but not the roof pitch or overhang – the 3D detail that really adds value.
Another thought might be to create 2D data by flattening the 3D data, but this also is not viable. Only a subset of the 2D data will be captured in 3D – typically, the buildings but not the streets, for example – and so any flattened 2D objects would need to be carefully integrated into the existing data to create cleanly connected geometries and maintain the existing identifiers. In addition, the 3D data captured with sensors might now be more positionally accurate than the historic 2D data that has been maintained over 50 or more years and so not trivial to integrate.
It seems inevitable then that the 2D and 3D datasets will need to be processed in parallel, but it can be overwhelming to deal with this master data management problem: synchronisation of data that is captured possibly using separate processes with different timescales. If we can make the synchronisation easier then not only does managing 2D and 3D in parallel become possible but it can also be used to benefit and enhance the quality and timeliness of the 2D data based on the 3D data, and vice-versa.
To deal with the quantity of data and the frequency of update, automating these processes is key. But to be successful and useful, the process needs to be context-sensitive and spatially intelligent enough to handle the inherent fuzzy differences between 2D and 3D representations.
How much difference can we tolerate?
An initial step would be to compare the 3D geometries with the 2D geometries to report on differences automatically. Applying an automated approach that compares the ‘flattened’ 3D with the 2D object will stumble over subtle differences in the geometry, due to differences in the capture process and the respective levels of detail. For example, a 3D model might include the overhanging roof or small buttresses that are not present in the 2D data – which does not include the roof overhang and has simplified away the buttresses. A comparison process would ideally be rules-based so that it can avoid false positives by ignoring these differences, while detecting genuine differences such as positional accuracy offsets, temporal differences (three buildings have been destroyed replaced by a single new one) and model differences. Model differences would be issues such as a 3D data representing a cluster of buildings as a single geometry, whereas the 2D data represents them as individual adjacent buildings.
Learning to live within the limits
Maintaining 2D and 3D data in parallel is not a perfect solution. It requires good data governance that tolerates some degree of difference in models. It does, however, enable data producers to apply the same amount of rigour to 3D data maintenance as they give to 2D. By accepting that neither can sit in isolation, we can ensure better harmonisation, provide quality assurance to users and gain confidence that the data is up to date.
The process needs to be rapidly applied every time either type of data is changed, so automation using rules makes this process efficient and repeatable, otherwise it is not viable to manage these differences manually. A rules-based automation process that can detect the true differences would also enhance the existing data management process in both directions. It will provide a new source of change intelligence in which the system can alert data managers to the features that have now changed in the real world and require updating. It can also drive automated correction such as applying positional accuracy improvements to the 2D data by automatically inferring and applying shifts based on matching to the equivalent features in the more accurate 3D data model.
For 1Spatial, that means using our no-code rules-based engine, 1Integrate, to verify, clean and synchronise data, leading to better national authoritative reference data that can be the launch pad for successful use of 3D in projects such as smart cities, environmental analysis, or even AR or VR use cases.
Summary
Ensuring harmonisation between 2D and 3D data is not therefore destined for discord, but it is challenging, with several possible approaches. Automation provides an answer to processing both types of datasets in parallel. By synchronising and making master data management easier, it enables data producers to improve the richness, quality and accuracy of their information and thereby realise its value. In doing so, it provides the justification needed to invest in the maintenance of 3D data to the same rigorous standard as 2D and can even be used to improve the quality of the 2D data.
Seb Lessware is CTO at 1Spatial (www.1spatial.com)