An Artificial Intelligence system developed at the Ecole Polytechnique Federale de Lausanne (EPFL) can produce 3D maps of coral reefs from camera footage in just a few minutes. Cécilia Carron reports on how this marks a major leap forward in deep-sea exploration and conservation capabilities for organisations like the Transnational Red Sea Center (TRSC).
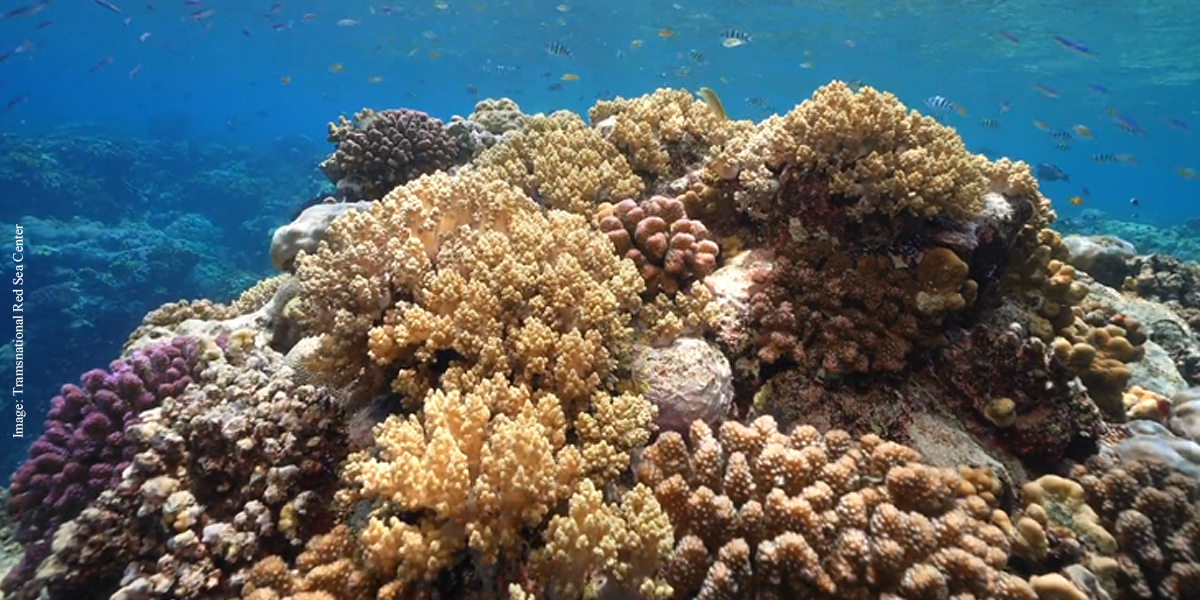
Corals often provide a colourful backdrop to photographs of shimmering fish captured by amateur divers. But they're also the primary focus of many scientists due to their ecological importance.
Corals—marine invertebrates with calcium-carbonate exoskeletons—are some of the most diverse ecosystems on Earth: despite covering less than 0.1% of the ocean's surface, they provide shelter and habitats for almost one-third of known marine species. Their impact also extends to human populations in many countries around the world.
According to research by the U.S. National Oceanic and Atmospheric Administration, up to half a billion people worldwide rely on coral reefs for food security and tourist income. But the world's corals are under threat from rising sea temperatures and local anthropogenic pollution, which causes them to bleach and die.
In response, organizations like the Transnational Red Sea Center (TRSC) are carrying out in-depth studies in an effort to unlock the secrets of coral species found in the Red Sea, which are uniquely resistant to climate-related stress. This EPFL-led initiative served as a testing ground for DeepReefMap, an AI system developed at the Environmental Computational Science and Earth Observation Laboratory (ECEO) within EPFL's School of Architecture, Civil and Environmental Engineering (ENAC).
The system can produce several hundred meters of 3D maps of coral reefs in just a few minutes from underwater images taken by commercially available cameras. It can also classify corals by recognising certain features and characteristics.
"With this new system, anyone can play a part in mapping the world's coral reefs," says TRSC projects coordinator Samuel Gardaz. "It will really spur on research in this field by reducing the workload, the amount of equipment and logistics, and the IT-related costs." The research is detailed in a paper published in Methods in Ecology and Evolution.
Local divers can easily capture data as they swim
Obtaining a 3D coral reef using conventional methods is not easy: costly, computationally intensive reconstructions are based on several hundred images of the same portion of reef of very limited size (just a few dozen meters), taken from many different reference points, and require the work of a specialist to obtain.
These factors severely limit the application of these methods in countries lacking the necessary technical expertise, and prevent the monitoring of large portions of reef (hundreds of meters, even kilometers).
But the AI-powered system developed at EPFL means data can now be collected by amateur divers: equipped with standard diving gear and a commercially available camera, they can swim slowly above a reef for several hundred meters, taking footage as they go. The only limits are the camera's battery life and the amount of air in the diver's tank.
In order to capture images over a wider area, the EPFL researchers developed a PVC structure that holds six cameras—three facing forward and three facing backward, located one meter apart—that can be operated by a single person. The apparatus offers a low-cost option for local diving teams, which often operate on limited budgets.
"A real revolution in the world of ecosystem conservation," says Guilhem Banc-Prandi, post-doctoral fellow at EPFL's Laboratory of Biological Geochemistry and Scientific Director of the TRSC.
Once the footage has been uploaded, DeepReefMap gets to work. This quick, agile system has no problem with the poor lighting, diffraction and caustic effects typical of underwater images, since deep neural networks learn to adapt to these conditions, which are suboptimal for computer vision algorithms.
Existing 3D mapping programs have several drawbacks. They work reliably only under precise lighting conditions and with high-resolution images. "They're also limited when it comes to scale: at a resolution where individual corals can be identified, the biggest 3D maps are several meters in length, which requires an enormous amount of processing time," explains Devis Tuia, a professor at ECEO. "With DeepReefMap, we're restricted only by how long the diver can stay underwater."
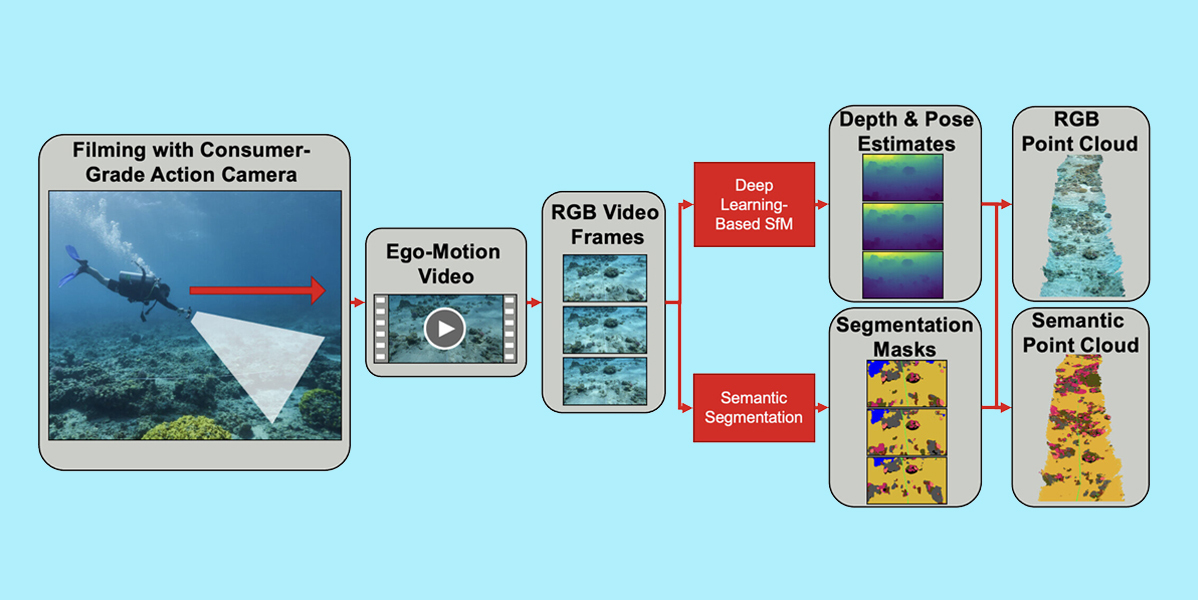
Categorising corals by health and shape
The researchers also made life easier for field biologists by including semantic segmentation algorithms that can classify and quantify corals according to two characteristics: health—from highly colorful (suggesting good health) to white (indicative of bleaching) and covered in algae (denoting death)—and shape, using an internationally recognized scale to classify the types of corals most commonly found in the shallow reefs of the Red Sea (branching, boulder, plate and soft).
"Our aim was to develop a system that would prove useful to scientists working in the field and that could be rolled out quickly and widely," says Jonathan Sauder, who worked on the development of DeepReefMap for his Ph.D. thesis.
"Djibouti, for instance, has 400 km of coastline. Our method doesn't require any expensive hardware. All it takes is a computer with a basic graphics processing unit. The semantic segmentation and 3D reconstruction happen at the same speed as the video playback."
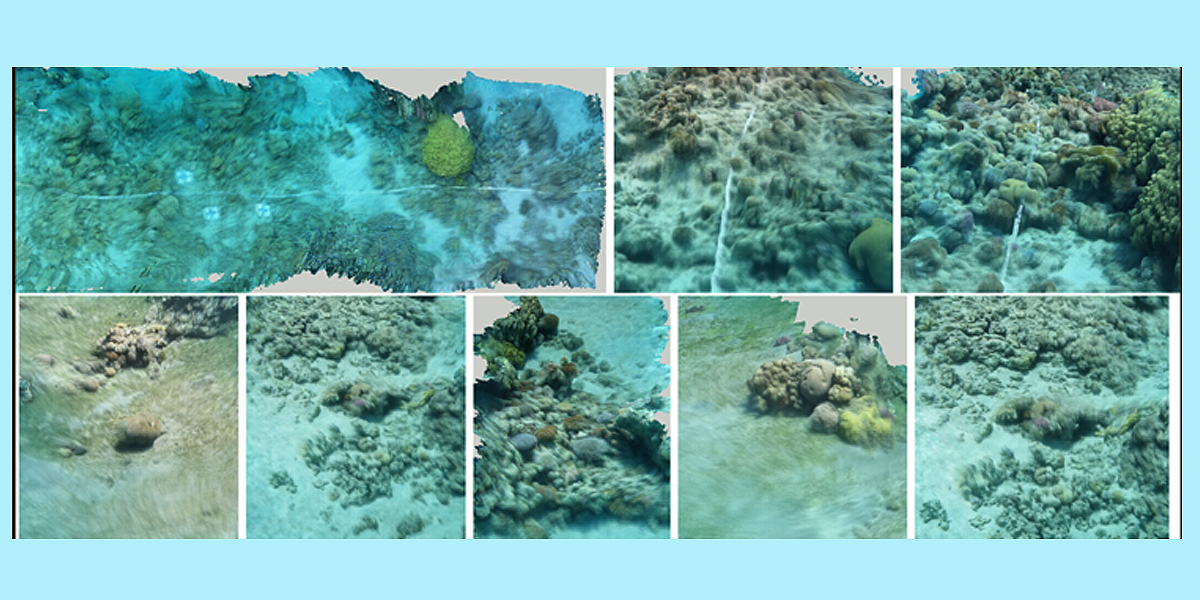
Toward a digital twin of the reef
"The system is so easy to implement that we'll be able to monitor how reefs change over time to identify priority conservation areas," says Guilhem Banc-Prandi, a postdoc at EPFL's Laboratory for Biological Geochemistry (LGB). "Having hard data on the abundance and health of corals is key to understanding temporal dynamics."
The new 3D mapping technology will give scientists a starting point for adding other data such as diversity and richness of reef species, population genetics, adaptive potential of corals to warmer waters, local pollution in reefs, in a process that could eventually lead to the creation of a fully fledged digital twin.
DeepReefMap could equally be used in mangroves and other shallow-water habitats, and serve as a guide in the exploration of deeper marine ecosystems. "The reconstruction capability built into our AI system could easily be employed in other settings, although it'll take time to train the neural networks to classify species in new environments," says Tuia.
More information: Jonathan Sauder et al, Scalable semantic 3D mapping of coral reefs with deep learning, Methods in Ecology and Evolution (2024). DOI: 10.1111/2041-210X.14307
Cécilia Carron is Scientific editor and press officer, Mediacom Content, at the Ecole Polytechnique Federale de Lausanne
Subscribe to our newsletter
Stay updated on the latest technology, innovation product arrivals and exciting offers to your inbox.
Newsletter